Spatio – temporal hotspot identification and evolution for COVID-19 in Pakistan
This project is aimed at early prediction of Parkinson’s disease through computerized analysis of online handwriting samples. While a number of clinical evaluations are carried out to diagnose the Parkinson’s disease, most of these tests are effective only once the disease is at a relatively advanced stage. Studies have shown that analysis of handwriting can be used as a valuable tool for prediction of Parkinson’s disease at very early stages. In the proposed research, we have employed raw signals captured by a digitizer tablet while attempting several writing and drawing templates, to compute various kinematic, temporal, and pen-based attributes. In addition to these online dynamic attributes, static offline visual features are also extracted using state-of-the-art Convolutional Neural Networks (CNNs). The developed tools allow practitioners to collect handwriting and drawing samples of subjects and employ a combination of different types of features (online and offline) for the analysis and prediction.

Disease data can be analyzed in context of spatio-temporal data as it includes both spatial and temporal information. A unique quality of ST data that differentiates it from other data studied in classical data-mining literature is the presence of dependencies among measurements induced by the spatial and temporal dimensions. For example, many of the widely used data-mining methods are founded on the assumption that data instances are independent and identically distributed (i.i.d.). However, this assumption is violated when dealing with ST data, where instances are structurally related to each other in the context of space and time and show varying properties in different spatial regions and time periods. Ignoring these dependencies during data analysis can lead to poor accuracy and interpretability of results. This research project aims at exploring predictive capabilities of multiple forecasting models using positive corona cases in multiple districts of Punjab, Pakistan. Better forecasting models directly impact public health policies, lockdown rules, vaccination policies and social lives in general. The evaluations are useful to understand the behavioral aspects influencing the frequency, peak and duration of disease outbreaks, that will allow better intervention strategies in order to prevent them or minimize the disease impact.
Publications
- Moetesum, M., Diaz, M., Masroor, U., Siddiqi, I., & Vessio, G. (2022). A survey of visual and procedural handwriting analysis for neuropsychological assessment. Neural Computing and Applications, 1-18.
- Diaz, M., Moetesum, M., Siddiqi, I., & Vessio, G. (2021). Sequence-based dynamic handwriting analysis for Parkinson’s disease detection with one-dimensional convolutions and BiGRUs. Expert Systems with Applications, 168, 114405.
- Moetesum, M., Siddiqi, I., Javed, F., & Masroor, U. (2020, September). Dynamic Handwriting Analysis for Parkinson’s Disease Identification using C-BiGRU Model. In 2020 17th International Conference on Frontiers in Handwriting Recognition (ICFHR) (pp. 115-120). IEEE.
Project Demo
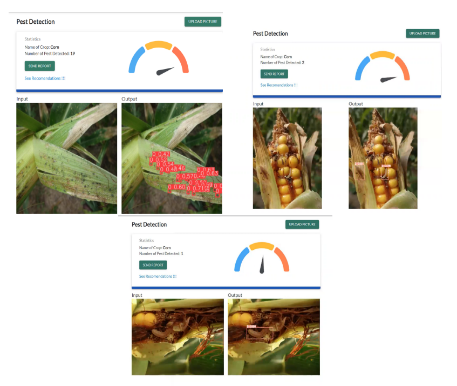
Project Demo
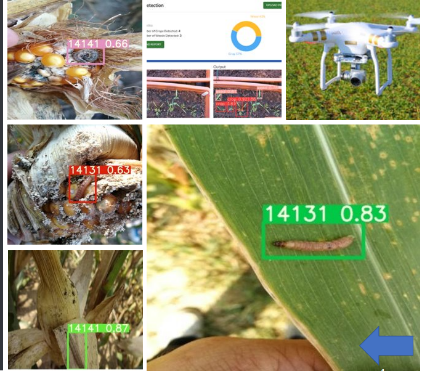
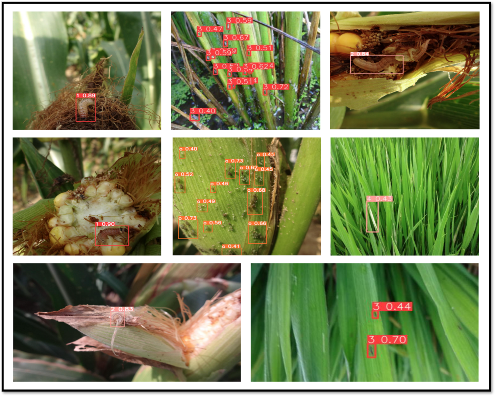