Carbon Footprint Analysis in Logistics using AI approaches for sustainable transportation
This project is aimed at early prediction of Parkinson’s disease through computerized analysis of online handwriting samples. While a number of clinical evaluations are carried out to diagnose the Parkinson’s disease, most of these tests are effective only once the disease is at a relatively advanced stage. Studies have shown that analysis of handwriting can be used as a valuable tool for prediction of Parkinson’s disease at very early stages. In the proposed research, we have employed raw signals captured by a digitizer tablet while attempting several writing and drawing templates, to compute various kinematic, temporal, and pen-based attributes. In addition to these online dynamic attributes, static offline visual features are also extracted using state-of-the-art Convolutional Neural Networks (CNNs). The developed tools allow practitioners to collect handwriting and drawing samples of subjects and employ a combination of different types of features (online and offline) for the analysis and prediction.
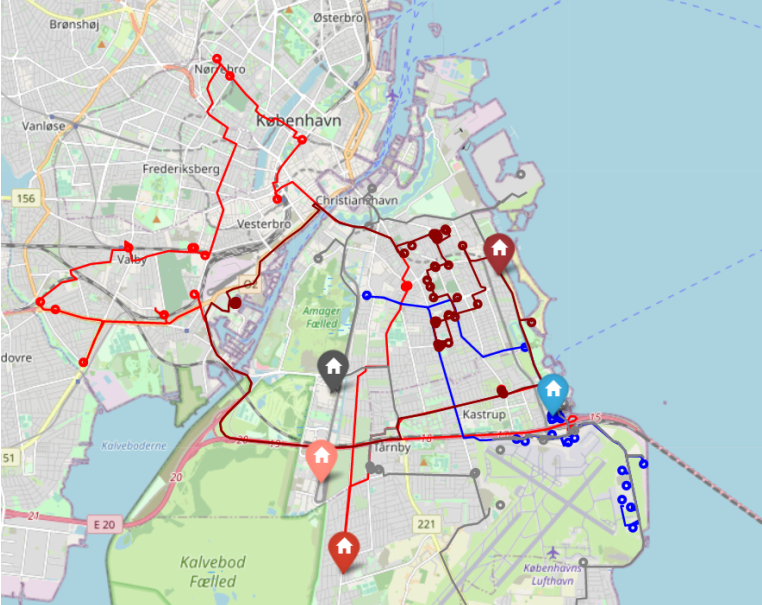
Carbon emission trading rules are standard practice for industrial corporations in developed countries. Corporations have to pay Carbon-tax that is used to low carbon emissions. Carbon-tax will ultimately result in an environment friendly industrial country. Carbon-tax makes an impact on industries to think about their carbon footprints. Consequently, industries themselves avoid carbon emissions. Carbon footprint is a measure of how much a person or an industry is producing carbon. This research uses classical algorithms (Farthest Insertion Algorithm) and AI based approaches, including SARSA and Q-learning to analyze the logistic routing problem at a reduced cost. Based on our analysis on logistic data, we hope to identify sustainable approaches that can reduce carbon emission providing logistics optimized carbon footprint systems.
Publications
- Moetesum, M., Diaz, M., Masroor, U., Siddiqi, I., & Vessio, G. (2022). A survey of visual and procedural handwriting analysis for neuropsychological assessment. Neural Computing and Applications, 1-18.
- Diaz, M., Moetesum, M., Siddiqi, I., & Vessio, G. (2021). Sequence-based dynamic handwriting analysis for Parkinson’s disease detection with one-dimensional convolutions and BiGRUs. Expert Systems with Applications, 168, 114405.
- Moetesum, M., Siddiqi, I., Javed, F., & Masroor, U. (2020, September). Dynamic Handwriting Analysis for Parkinson’s Disease Identification using C-BiGRU Model. In 2020 17th International Conference on Frontiers in Handwriting Recognition (ICFHR) (pp. 115-120). IEEE.
Project Demo
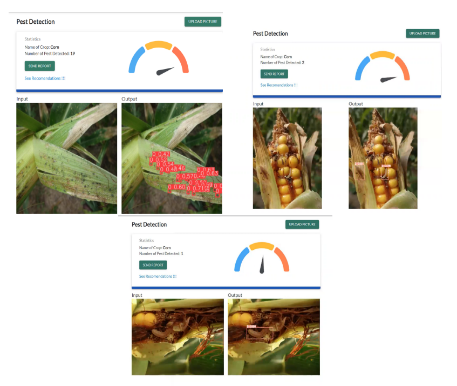
Project Demo
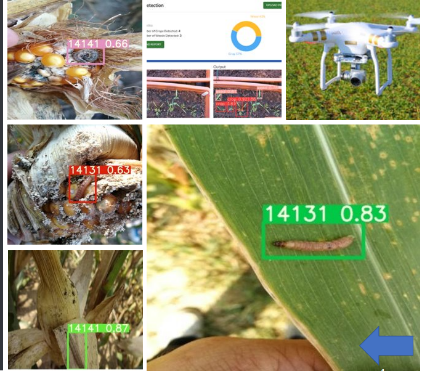
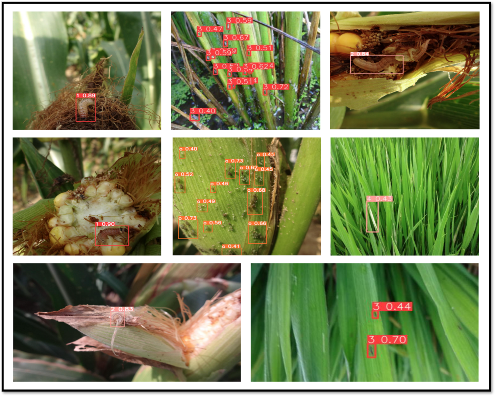